Introduction
In tandem mass spectrometry-based proteomics, proteins are digested into peptides by specific protease(s), but generally only a fraction of peptides can be detected. To characterize detectable proteotypic peptides, we propose a bidirectional long short-term memory (BiLSTM)-based algorithm, named DeepDetect, for the prediction of peptide detectability enhanced by peptide digestibility. Compared with existing algorithms, DeepDetect is featured by its improved prediction accuracy for a wide range of commonly used proteases, covering Trypsin, ArgC, Chymotrypsin, GluC, LysC, AspN, LysN, and LysargiNase. As an application, DeepDetect can be used to reduce the in silico predicted spectral libraries in data-independent acquisition mass spectrometry data analysis. Our experiments using DIA-NN software showed that DeepDetect can significantly accelerate the library search without loss of peptide and protein identification sensitivity. This tool is implemented in Python (v3.5.2) using Keras (v2.2.4, https://keras.io) with TensorFlow32 (v1.10.0) backend. Binary cross entropy is the loss function, binary accuracy is the metric, and Adam with default parameters is the optimizer.
Download
DeepDetect is written in Python, and is publicly available for non-commercial uses.
- DeepDetect (PyTorch) V1.0.0
- Source code (PyTorch)
- DeepDetect (TensorFlow) V1.0.0 (2023.2)
- Source code (TensorFlow) (2023.2)
- Data sets
Publications
- Yang, J.; Cheng, Z.; Gong, F.; Fu, Y. DeepDetect: Deep Learning of Peptide Detectability Enhanced by Peptide Digestibility and Its Application to DIA Library Reduction. Anal. Chem. 2023, 95, 15, 6235–6243. https://doi.org/10.1021/acs.analchem.2c03662.
- Yang, J.; Gao, Z.; Ren, X.; Sheng, J.; Xu, P.; Chang, C.; Fu, Y. DeepDigest: Prediction of Protein Proteolytic Digestion with Deep Learning. Anal. Chem. 2021, 93 (15), 6094–6103. https://doi.org/10.1021/acs.analchem.0c04704.
Contact
Address: No.55 Zhongguancun East Road, Haidian District, Beijing, China
Postcode: 100190
Any problem with the software or this website, please contact:
Yan Fu's Research Group
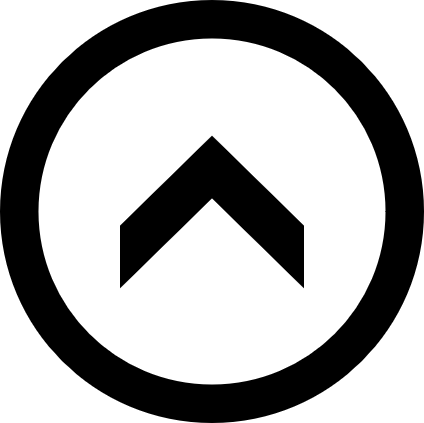