Introduction
Proteolytic digestion of proteins by one or more proteases is a key step in shotgun proteomics, in which the proteolytic products, i.e., peptides, are taken as the surrogates of their parent proteins for further qualitative or quantitative analysis. Generally, the proteases cleave proteins at specific amino acid residue sites, but digestion is hardly complete (wide existence of missed cleavage sites). At present, systematic studies about the commonly used proteases in proteomics are insufficient, and there is a lack of easy-to-use tools to predict the cleavage sites of different proteases. Hence, we propose a novel sequence-based deep learning algorithm -- DeepDigest, which integrates convolutional neural networks and long short-term memory networks for protein digestion prediction. DeepDigest can predict the cleavage probability of each potential cleavage site on the protein sequences for eight popular proteases including trypsin, ArgC, chymotrypsin, GluC, LysC, AspN, LysN, and LysargiNase. Compared with three traditional machine learning algorithms, i.e., logistic regression, random forest, and support vector machine, DeepDigest showed superior performances for all the eight proteases.
Download
DeepDigest is written in Python. Please click the link below to download the command line tool:
DeepDigest V1.7.0 (2023.2)
Notes:
--- This is a command line tool for eight proteases (Trypsin, ArgC, Chymotrypsin, GluC, LysC, AspN, LysN, and LysargiNase). Please choose the consistent model for prediction.
--- There are the user guides ( in English / in Chinese) for this command line tool.
--- Download the test dataset here.
Publication
- Yang, J.; Gao, Z.; Ren, X.; Sheng, J.; Xu, P.; Chang, C.; Fu, Y. DeepDigest: Prediction of Protein Proteolytic Digestion with Deep Learning. Anal. Chem. 2021, 93 (15), 6094–6103. https://doi.org/10.1021/acs.analchem.0c04704.
Contact
Address: No.55 Zhongguancun East Road, Haidian District, Beijing, China
Postcode: 100190
Any problem with the software or this website, please contact:
Yan Fu's Research Group
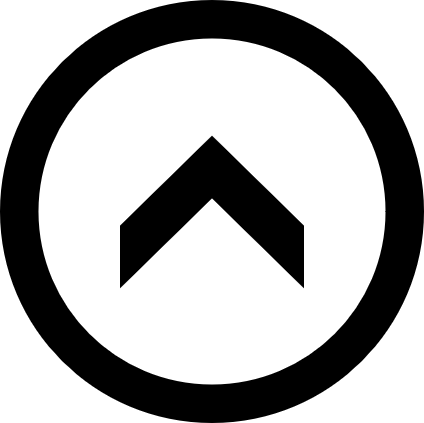